Better Results with Domain-Specific AI for Sustainable Business & Finance
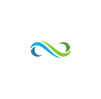
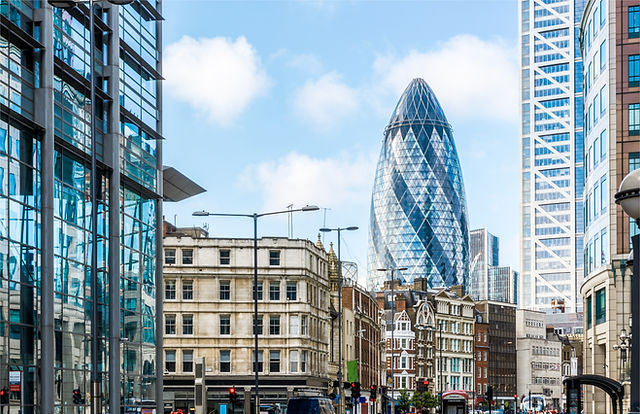
Better Results with Domain-Specific AI
for Sustainable Business & Finance
FP&A In Focus
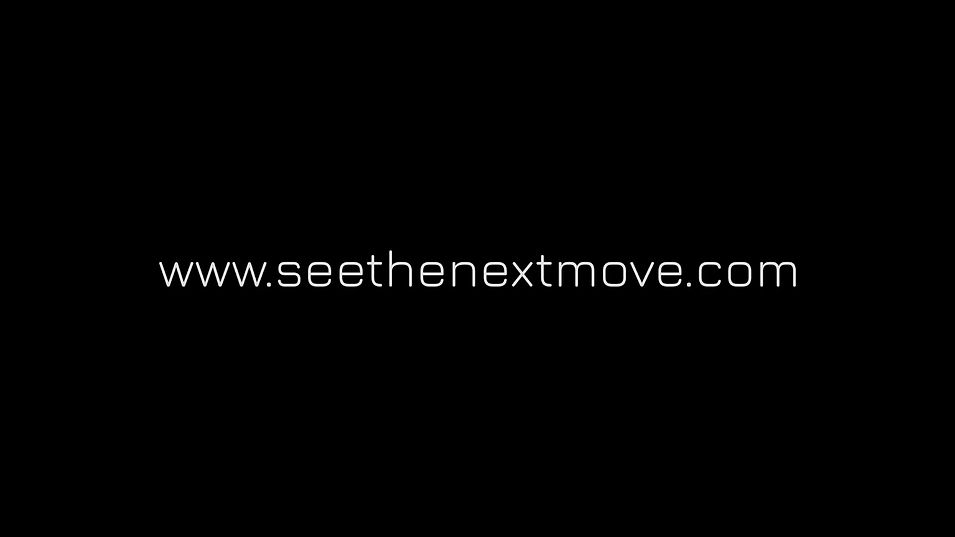
FP&A + AI = XP&A + 3 Ps
The world of Business & Finance is changing rapidly, and with it, the role of the Financial Planning and Analysis (FP&A) function is evolving as well. The traditional FP&A function focused on budgeting, forecasting, and financial analysis, requires a better value proposition as today's fast-paced business environment demands more from finance professionals. That's why we're seeing a shift towards a new type of function: the Extended Planning and Analysis (XP&A) function, focused on the triple bottom line (i.e. Profit + People + Planet.)
​
The shift towards XP&A, empowered by AI, is a testament to the growing importance of ESG principles in the corporate world. This holistic approach to financial planning and analysis ensures that companies do not merely survive but thrive by balancing economic performance with social responsibility and environmental integrity. As we advance, the role of XP&A professionals will be pivotal in steering businesses towards a future where profit, people, and the planet are in harmony, securing a legacy of prosperity and stewardship for generations to come.
​
The use of advanced Forecasting Techniques as well as technology is critical to support the above and our clients are increasingly focusing on critical algorithm selections:
​
-
ARIMA - Autoregressive Integrated Moving Average
-
Description: A statistical analysis model that uses time series data to forecast future points. ARIMA models are characterized by their use of differencing of observations (integrated part), autoregression (AR part), and a moving average (MA part) to predict future values based on past values.
-
-
LSTM - Long Short-Term Memory
-
Description: A type of recurrent neural network (RNN) specifically designed to avoid the long-term dependency problem. LSTM networks are capable of learning order dependence in sequence prediction problems. This makes them particularly useful for forecasting tasks where the context from long-term patterns is crucial for making accurate predictions.
-
​